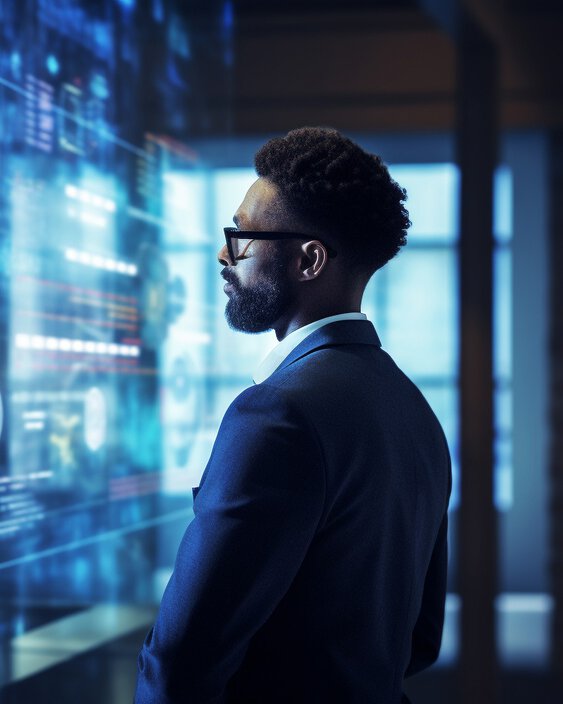
L&H data analytics services: hr | bluebox
In our industry, data has always formed the foundation of business operations. The emergence of new possibilities has unlocked a world of opportunities. The true value of these can be unlocked through professional analysis. An in-depth understanding of data allows for the reduction of risks, the pricing and selection of profitable business ventures and the active management of portfolios. Data analytics empowers you to achieve a positive impact on your balance sheet through strategic data-driven insights.
Loading ...
Untapped opportunities lie within your data. We are here to help you leverage it effectively.
Links
Leveraging our expertise enables risk-appropriate offerings at fair prices to consumers. Our data analytics capabilities allow swift adaptation to market changes, seizing opportunities and optimising costs. Partnering with us grants access to hr | bluebox, our global data analytics service.
We support you in drawing the right conclusions from your data to form an improved understanding of your portfolio, as well as consumer behaviour and motivation in your market. In particular, our data analysts work hand-in-hand with the business development, market representatives, valuation and risk management teams at Hannover Re to identify risks and opportunities for you.
"Consultancy-wise hr | bluebox is a slam dunk"
What is hr | bluebox?
hr | bluebox is not a machine solution but a project-driven data analytics service that helps you make better decisions today about tomorrow’s opportunities.
Insurance companies collect valuable data for strategic decisions, and our hr | bluebox service enables reinsurance clients to uncover patterns, trends, and connections within their data for actionable strategies.
Our streamlined hr | bluebox data analytics service delivers top-quality insights into, for example, your application, portfolio and claims data using a combination of statistical tools and Artificial Intelligence techniques – which we optimise for your business – together with personalised interpretation and advice from our analysts. It’s a two-way process, requiring human commitment from you and us.
What makes hr | bluebox special?
With our tools and strategies, we generate insights traditional methods fail to find. Our data scientists add value by scrutinising and enriching your data. Our advanced Artificial Intelligence techniques identify meaningful patterns, allowing us to work with you to suggest possible ways to address your needs. We add the human touch by:
- Checking and improving data quality
- Tailoring machine learning solutions to fit your individual needs
- Translating the results into tangible business actions
- Evaluating the impact of these actions
Let us help you unlock the opportunities in your portfolio data with hr | bluebox: our all-inclusive data preparation, visualisation and strategy-based service for tomorrow’s insurers. We add the human touch.
How does hr | bluebox help?
Unlike third-party providers, our interests are directly aligned with our clients' success and the overarching goal is always to use data insights to improve their business. In past projects we addressed topics such as retention management, cross- and upselling, accelerated underwriting, claims analysis as well as Machine Learning model validation.
Examples of how our global hr | bluebox service has benefited clients include a project where we enhanced a client's automated underwriting model using interpretable AI to significantly reduce their medical underwriting costs. Another example is our work in lapse analysis, where we have helped clients avoid unprofitable commission expenses by identifying early lapses and recognising loyal customers. Furthermore, we predicted future lapses using explainable AI which allowed our clients to employ prevention measures. We have also helped clients identify high performing, as well as problematic and fraudulent sales agents and monitor the impact of key market indicators on customer behaviour.
Our commitment to honouring the unique characteristics of various markets, products, and business needs is evident in these sample cases. Get in touch to explore the ideal solutions for your business needs!
"Explanations were great, especially adding value with ML methods […]"
Unleashing the power of your data together
Through collaboration across teams and close client partnerships, we unlock the full potential of data analytics to deliver smarter solutions, deeper insights, and tangible impact. At a recent life & health offsite, our experts shared their perspectives on how data analytics creates value where it matters most.
Dr. Yinglin Zhang on data analytics: Insights and opportunities
Data is hailed as 'the new gold', and life & health insurers have access to a wealth of it. However, it is only through professional analysis that its true value can be unlocked. This insightful interview with Dr. Yinglin Zhang delves into the world of data and explores the transformative power of data analytics for insurance companies: